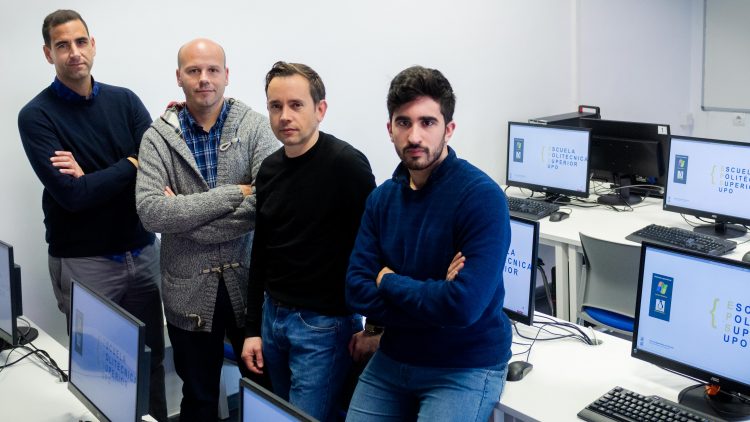
Researchers from the Universidad Pablo de Olavide (UPO), liaised with researchers from the Universidad Americana from Paraguay, have carried out an in silico study, i.e. a computer simulation study. Biomedical data of 187 patients have been used to identify new genetic biomarkers, which are involved in tumour development of lung cancer. The leader of the multidisciplinary team is the professor Francisco A. Gómez and it is composed by researchers from the Intelligent Data Analysis Group and from Data Science & Big Data Research Lab (UPO), including Fernando M. Delgado, Miguel García and Federico Divina.
The aim of this project is the creation of in silico mathematical models of the genetic interaction by using computational and high-level artificial intelligence techniques. Specifically, this model is called ‘gene network’ and tries to visually represent the interactions among different types of genes of an organism for a specific biological process. The gene networks are presented visually as a graph, in which the different genes are connected considering their interactions. Thus, it may be said that the gene networks represent a ‘graphic’ of the diverse types of gene interaction in certain process or diseases.
Once generated, the analysis leads to a better understanding and study of the molecular and cell mechanisms, which are both involved in the disease development of the lung carcinoma in this case. Specifically, this model means an integrative approach to the massive study of the gene expression, which considers the gene cluster of the patients involved in the tumour development. “Our understanding about how genes interact among them has been enhanced due to these approaches, which can carry out the analysis of thousands of genes simultaneously”, reported Francisco A. Gómez.
However, the task of generating these models is usually tough to undertake through a traditional experimental work performed in the laboratory, due to the huge volume of gene information which must be studied. For this reason, the new computational techniques of artificial intelligence and machine learning have emerged as a solution to reduce costs and to delimit laboratory experiments and study completion dates.
This work accomplished is particularly relevant, as lung carcinoma is one of the most aggressive cancers and has the worst prognosis, mainly due to its late diagnosis. The expectation of full recovery after treatment is a devastating prognosis, even in the early stages of the disease. Because of this, considerable efforts are being made to get an early diagnosis, which could be helpful for the patient to be optimistic. In this context, biomarkers are a sensitive early diagnostic tool. These biomarkers are substances or molecules which are obtained through a non-invasive procedure, e.g. blood sample, and they are also an indication of the presence of tumour process. Apart from the diagnostic purposes, the biomarkers can be used to establish the stage of the disease and predict the patient’s response to a treatment. “These biomarkers are key elements to progress in fighting against diseases like cancer”, said Francisco A. Gómez.
The first outcomes achieved have been recently published on the genetic specialised journal Genes, showing a set of biomarkers identified in a substantial proportion of clinical cases. While the preliminary data require a further experimental development in the laboratory, the outcomes obtained are encouraging, since the underlying molecular mechanisms of this disease can be deeply defined through these tools in order to develop a therapeutic approach subsequently.
Network models applicable to the study of other diseases
The technology used can be applied to many clinical cases since, apart from being useful for the lung carcinoma study, these models of gene networks have proved to be useful for studies of various diseases, such as mental health diseases. For instance, these network models can be applied to the post-traumatic stress study, according to another recent work coordinated by Francisco A. Gómez, also in cooperation with Fernando M. Delgado, Miguel García and Federico Divina.
In the last-mentioned research, the models of gene networks generated in silico were useful for distinguish genetically between two groups of patients: one that showed signs of post-traumatic stress and other without evidence of mental disorder.
Full article about in silico models used for identifying gene biomarkers involved in lung cancer:
Delgado-Chaves, F.M.; Gómez-Vela, F.; García-Torres, M.; Divina, F.; Vázquez Noguera, J.L. Computational Inference of Gene Co-Expression Networks for the identification of Lung Carcinoma Biomarkers: An Ensemble Approach. Genes 2019, 10, 962.
https://doi.org/10.3390/genes10120962
Full article about models of gene networks applicable to the post-traumatic stress study:
Gómez-Vela, F.; Delgado-Chaves, F.M.; Rodríguez-Baena, D.S.; García-Torres, M.; Divina, F. Ensemble and Greedy Approach for the Reconstruction of Large Gene Co-Expression Networks. Entropy 2019, 21, 1139.
https://doi.org/10.3390/e21121139